Benefiting From Testing in the age of AI
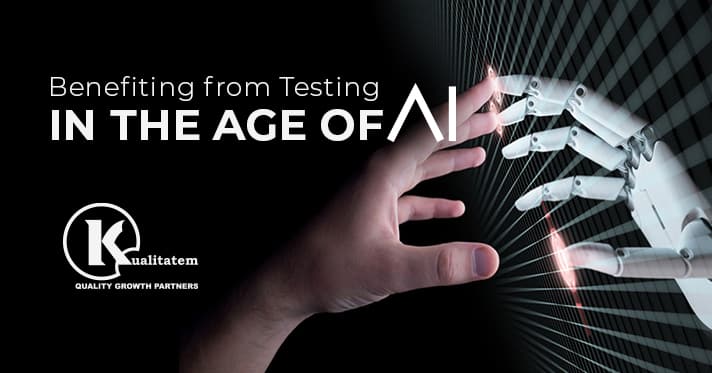
- January 21, 2020
- HibaSulaiman
With countless opportunities that technological advancements have made possible at the forefront, running your own business has never been easier. However, to succeed, it is vital to set yourself apart from your competitors and increase your Return On Investment (ROI). Without any doubt, software applications are complex technological systems that developers have constructed. The rapid development and complexity of software applications call for sophisticated quality assurance checkpoints during various stages of the Software Development Life Cycle (SDLC). Software engineers partnering with an independent software testing company are identifying new mechanisms to ensure the quality and reliability of software systems. Artificial Intelligence (AI) is making its way forward by changing the software testing techniques and processes.
However, enterprises are still unclear about what to expect when it comes to the ROI of AI. Most of the software engineers believe that AI is just like any other software solution. But industry leaders are of the view that testing has become much more efficient with AI-powered software testing tools. AI makes it easier to optimize a website through testing and analytics. It is mostly based on guesswork but utilizes advanced testing and metrics. But identifying reliable metrics can be a challenging task.
Measuring Artificial Intelligence ROI
There is a lot of uncertainty and experimentation involved in AI projects before they can achieve success. Industry leaders believe that the identification of a measurable metric for a projected return may be relatively simple. For instance, by using predictive maintenance applications in the manufacturing sector, businesses can link directly to a reduction in maintenance costs. But when talking about other applications like improving customer experience in the retail sector, identifying a small number of reliable metrics to measure ROI is more challenging.
Thus, businesses need to have a clear understanding of the returns, otherwise, they may risk losing their investments in AI. One possible way to ensure having a measurable metric is to choose a specific business issue where a non-AI solution is being used, measured and tracked. Let’s have a look at all opportunities enterprises can tap for software quality assurance by using Artificial Intelligence (AI) and Machine Learning (ML):
Enhancing Software Quality
Software quality is a customer’s perception of how well an application performs in use. Reliability, performance, and usability are intrinsic factors that define software quality and is one of the biggest challenges for enterprises. Testing software apps and systems is a cumbersome process and thus requires the use of AI. AI can be used in generating tests, reducing repetitive analysis and using production data. AI can also help in tracking features to distinguish what to automate and what to test.
Automating Test Designs
AI can also generate test cases from the user requirements to provide maximum coverage. This may not be a feasible idea in the future because test cases are currently derived from requirements that are explained in natural language. So AI should be able to understand both, requirements in detail and also its context, meaning, and relevance to estimating the risk contribution of that requirement. AI should also be capable of linking outcomes from these learnings to the app’s technical components so that testers can derive test cases from the given requirements. Thus, industry leaders should explore AI capabilities for automated test designs in the future.
Preventing Redundant Test Cases
It is critical to identify identical test cases physically, but that is not a solution to prevent redundancy. Identifying identical test cases logically is much more challenging and time-consuming. Currently, it is not an economical solution to train systems to detect these automatically. Testers need to flag the business relevance of test data appropriately. Once they have achieved this, it is possible to eliminate bottlenecks and avoid redundant test cases.
Maximum Risk Coverage
AI can be used to maximize business risk coverage and defect detection to optimize test execution – especially for independent software testing company. Since enterprises have limited time, resources and budget constraints, mathematical algorithms can be used to achieve success. It involves maximizing defect detection, reducing costs, and the number of test cases. It is important to find out the probability that a certain test case will detect a defect or not. So that this strategy can work successfully. Testers can set this probability by approximating from past test runs. It is also crucial to find out the risk contribution of each individual test case. Testers also require knowing the average execution time for each test case which can also be derived from the past test runs. In the case of new test cases, the time can be estimated based on the average execution time of test cases with similar sequences. Once all this information is available, AI capabilities can be leveraged to provide maximum risk coverage.
Miscellaneous Test Cases
When an independent software testing company leverages AI in software testing, there are other possible use cases that can improve business ROI including:
Portfolio Inspection – AI can also track unused test cases or test cases that are not linked with requirements
Automated Exploratory Testing – AI can also interact with the application, reveal defects and automatically extract test cases to reduce regression testing efforts.
False Positive Detection – AI can also display results by highlighting if a failed test case affects an application negatively or it is just a result of technical issues caused by a developer.
Automated Defect Diagnosis – AI can highlight potential reasons as to why a test case failed to reduce the efforts and time taken in determining the main cause of failure.
Conclusion
Currently, Artificial Intelligence (AI) and Machine Learning (ML) technologies are contributing a great deal to the software industry. Most of the technological challenges faced by an independent software testing company can be met by leveraging AI while improving the efficiency of testing processes and increasing the ROI for businesses.