Building Fundamentals Before Leveraging Artificial Intelligence
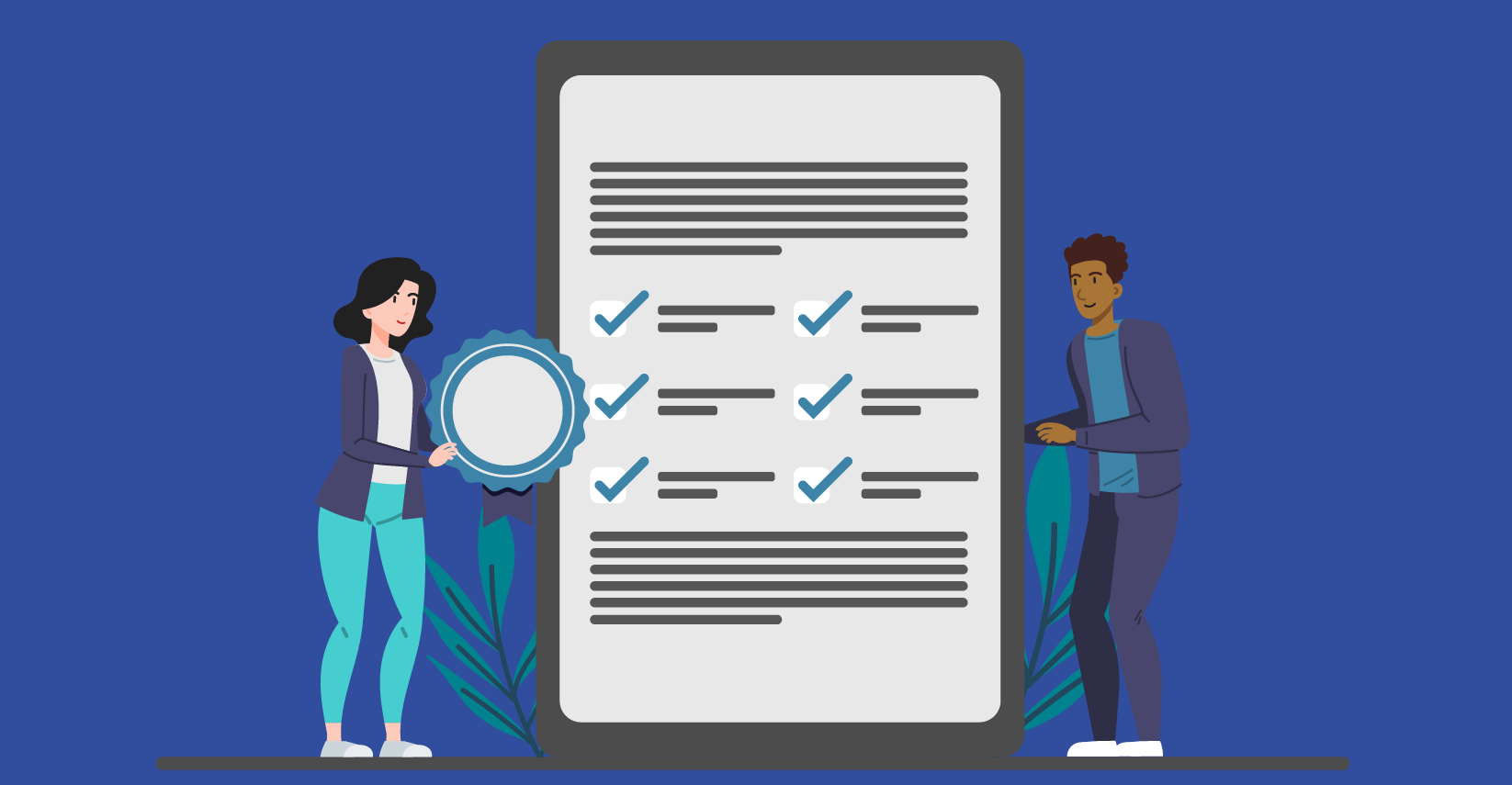
- June 3, 2024
- Malaika Saeed
Integrating artificial intelligence in any industry reshapes its landscape, whether it is software development or quality assurance. Without any doubt we can say that AI has enhanced the efficiency of and results of these fields.
However, there is a strategic approach to everything even to integrate AI in anything either it is automation testing or other QA processes.
For any software testing company or QA service provider, it is necessary to build a strong foundation in traditional quality assurance before going for AI.
Let’s learn why it is essential to have solid fundamentals for effectively using the power of AI in software testing.
The Importance of a Strong QA Foundation
Quality assurance is an essential component in bringing quality to software development. It makes sure that the softwares meets customer demands and also delivers a better user experience. It must be an initial focus for a QA software testing company to establish a reliable QA process.
This includes the development of comprehensive testing methods, rigorous execution processes and detailed test plans which were designed to detect defects at every stage of software development.
A well-structured quality assurance procedure ensures systematic testing coverage, which includes functional and usability testing as well as performance and security checks.
Each of these testing aspects necessitates rigorous attention to detail and an organized strategy to guarantee that the software functions consistently under a variety of scenarios.
Test Automation: A Crucial Step Before AI Integration
Automated software testing is a vital part of effective development projects because it improves the efficacy, efficiency, and accessibility of software testing.
Manual testing entails humans interacting with program interfaces, comparing results to intended behavior, and documenting findings.
Automated testing programs can replay previously recorded events, compare them to predicted behavior, and signal success or failure. Automated tests can be conveniently repeated and expanded to perform things that would be impossible to complete manually.
It saves both money and time by cutting the time required to execute repeated tests from days to hours. It can also enhance accuracy by repeating the same actions exactly and capturing detailed findings. Automated testing can improve test coverage by running hundreds of complicated test cases with each test run.
Automation can replicate tens, hundreds, or millions of virtual people communicating with a network, software, and websites, saving developers time and boosting trust. It also benefits testers and developers by detecting issues promptly before they are sent to QA, enabling them to concentrate on more difficult projects.
Thus before getting AI integrated, it is essential for test automation companies to have a solid automation framework that can handle complexities. These complexities can be associated with rigorous application testing. Having a strong test automation foundation that is strong can make a company ready to have AI incorporated effectively to improve testing capabilities.
Integrating Artificial Intelligence in the QA Process
Artificial intelligence software testing improves accuracy and speed by reducing human mistakes, allowing testers to concentrate on more important duties. AI can also simplify automation by automating complex procedures using natural language commands, removing the need for time-consuming coding. It also offers complete coverage by testing every possible test case, resulting in high test coverage in little time.
AI tools can automate repetitive tasks like automated test case creation, test case prioritization, intelligent test data generation,regression suite automation, test automation,, defect prediction, performance testing, security testing, natural language processing (NLP), visual testing, predictive analysis, continuous testing and integration, chatbots for user interface testing, behavior-driven development (BDD), and auto-healing locators. These solutions offer proactive, mitigating, and continuous testing throughout the development lifecycle, ensuring a smooth execution.
AI in Automation: Enhancing Capabilities, Not Replacing Them
AI has spurred controversy over its ability to replace human jobs in a variety of industries, particularly information technology (IT).
However, an additional point of view implies that AI can supplement and improve the talents of IT workers instead of replacing them. AI solutions can boost productivity as well as efficiency by automating regular processes, allowing IT workers to concentrate on more difficult and strategic duties.
AI systems can also help with problem solving by evaluating trends and patterns in massive datasets, detecting flaws or potential hazards that humans may miss.
Technologies with integrated AI can offer useful data and suggestions for decision-making in areas such as allocation of resources, project planning, and technology investments.
AI-powered systems for monitoring can enable around-the-clock monitoring of IT infrastructure, allowing for quick reactions to manage risks and reduce downtime.
AI enables skill upgrading and upskilling, fostering continual learning and progress among the IT workforce. AI solutions can assist enterprises in lowering operational expenses and increasing the life span of IT assets by automating mundane tasks and streamlining procedures. The incorporation of AI into IT workflows fosters innovation, resulting in a brighter, more technologically sophisticated future.
The Risks of Skipping Fundamentals
Inadequate Testing Coverage
Traditional testing typically covers the critical edge cases, but AI driven tests might overlook them sometimes. As a result, the software tested has unaddressed vulnerabilities that can affect user experience later and automatically lead to the poor performance.
Poor Quality Data
AI systems need clean, thorough, and organized data to learn properly. Data of poor quality can result in algorithms that are ineffective or erroneous.
Increased Costs
If the companies don’t pay attention to establish fundamental QA practices they might need to repeatedly correct the preventable errors. This will ultimately increase project costs.
Loss of Human Insight
Experienced human testers can catch critical issues which Artificial intelligence can miss. The other problem is AI lacks the complete understanding of context that human testers usually give.
Strategies for Effectively Integrating AI in QA
Integrating AI to QA testing is beneficial but it would need effective strategies that can help to enhance the workflow and improve productivity. Here are some strategies given below:
AI Powered Test Case Generation:
Bringing AI integrated tools in the use to generate test cases can be a useful strategy. These tools automatically generate the test cases on the instruction provided by the user. This improves the test coverage and reduces manual efforts.
AI-Assisted Defect Management
Defect management can be improved with AI integrated test management tools. They enhance defect detection and identify anomalies at every stage. Thus we can say it leads to reduced manual efforts.
AI-Based Test Data Generation
Utilizing artificial intelligence to generate test data helps testers by reducing their efforts for manual data creation.
AI-Enhanced Test Case Maintenance
Utilize AI to maintain and update test cases, ensuring they remain relevant and effective over time.
Set KPI’s
Set key performance indicators (KPIs) to assess the effect of AI on the QA process, such as shorter testing times, higher defect detection rates, and better test coverage.
Conduct regular assessments of the return earned from AI integration, taking into account both quantitative metrics (such as time and cost reductions) and qualitative elements (such as increased test accuracy and team efficiency).
Bottom Line
It’s an outdated technique if you still use traditional QA testing methods only and still do all the tasks manually. Integrating AI in the testing can bring you ease and help you shift your workload to AI.
But setting up essential fundamentals before integrating AI in the testing is necessary to have better use of AI for testing. So set fundamentals before leveraging AI for QA testing.